Tell me a bit about what you do.
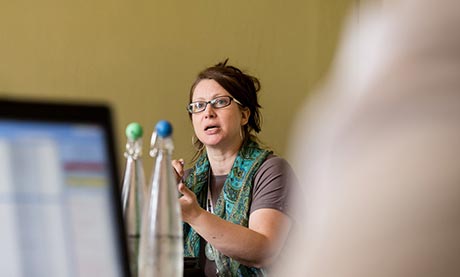
I’m based in Bangkok at the Mathematical and Economic Modelling Unit (MAEMOD), which is part of the Mahidol Oxford Research Unit (MORU). The main work that we do is economic-epidemioloigcal modelling with a focus on mechanistic approaches, and we also do enhanced data analysis.
A nice thing about having a modelling unit embedded in a larger tropical medicine research group is that our work is closely linked with other research. We start producing the models quite early in the process – often before the data are collected. This establishes a feedback loop: writing a model for a particular system raises questions about how the system works and quite often uncovers gaps in the knowledge that can be easily filled in with experiments or field work.
So, modelling can help inform study design – for example, what data to collect or where and when to collect it?
Absolutely. For us, it means that we can request data that wouldn’t have been part of the original design – and just a little tweak can mean that we get a more complete picture of the biological system. And, then after the fact, because we collaborate with different people within MORU and outside, the models can be used to combine lots of different data sets, creating a framework that gives a 360° view of the system using all the data available rather than just from a particular study.
How can this framework help people to understand the implications of a particular public health intervention?
That was the other part of the puzzle that I wanted to talk about. Once the models are set up and fully validated against data sets, they can be used to project into the future to answer ‘what if’ questions about public health interventions. If you change your public health policy, what sort of impact can you expect to see? What if you don’t change it? Things don’t carry on in the same way if you don’t change. The main disease that we’re studying lately is falciparum malaria and with artemisinin resistance, for example, if you don’t change your policy, our models are predicting that the cases and the mortality rates aren’t going to remain constant. Model projections allow you to consider multiple scenarios in the future. They’re not perfect of course; they can’t perfectly predict the future. No modeller would say that they could.
Not a crystal ball.
No, but that’s related to how we got our name. Our Thai colleagues suggested MAEMOD, which is an abbreviation of mathematical and economic modelling—and also happens to be the Thai word for witch. One idea being that witches can see into the future. That’s all in good fun. Of course, what models actually do is extrapolate. They’re probably the best framework to extrapolate what you have now – all of the information you have now – put together into the future.
All the best models will not only give you a single line prediction but will give you a prediction interval, so you’ve got that uncertainty. Returning to the artemisinin resistance example, the bottom end of the confidence interval is saying, ‘Meh, no change’ – cases may remain constant – but then the upper end is predicting quite large increases in incidence, in mortality and morbidity. We try to predict the most likely outcome but also the full range of what might happen based on what we do and don’t know, right now.
How do models deal with unknowns?
A nice thing about models is that you can run them thousands and thousands of times. This is particularly useful if you don’t know what a parameter is or you don’t know something about a system but you think that it might be important. A huge unknown for malaria is the way that populations are linked in terms of their transmission – it’s a black box – and this is an area where I think that genetic information can help.
How do you go about putting together a model?
The point of a model is that it simplifies the real system, so I always start as simply as I possibly can and see if I can answer the question that I’ve been asked. I only go to more complex structures if I have to. That’s my approach. Other modellers have a different approach. The nice thing about working with other modellers is you can say, “I got this with my model, your model’s far more complex – it’s got other things in it – did you get the same answer?”
That’s important because all models have simplifying assumptions. There isn’t one right model. There are wrong models, but there isn’t one perfect model. So it’s always good, especially with results that have important public health policy implications, to seek confirmation from other models that have used different assumptions.
Has your simplified approach helped people to understand what modelling can do and how it relates to their own work?
Interestingly, it’s a double-edged sword. The simple models are very easy to explain to people but they don’t like them. Especially people who are out in the field doing their medical research, doing their interventions, because of course it’s not as simple as that.
It’s good to engage in a conversation [with stakeholders] to find out what they think is the most important thing to have in the model, because if you try and reproduce everything you’ll still be doing it ten years down the line. If you need an answer in a year’s time or a week’s time, then you have to use a simple model structure and to give your answer with all the health warnings that go with it, which is to say – it was a simple model structure, we didn’t have this in it, we didn’t have that in it, etc. The more complex the model, the longer it takes to run. Some models you can run on a laptop, some you can solve by hand on a piece of paper, and some require a computer cluster.
How do you engage stakeholders in these conversations?
The way we started – and we continue to work – is that we engage with policy makers by first having a one-day, introductory workshop on mathematical modelling and economic modelling. The point of that workshop is to invite members of NMCPs [National Malaria Control Programmes], other policy makers and potential collaborators, and also potential trainee modellers to find out about modelling, to learn how it might be useful for them – basically how they might access that kind of technique and capacity to support their policy making.
I take it that capacity building is an important component of your work?
Capacity building is one of my main motivations, apart from research. I really feel very strongly that it’s so important. And particularly for modelling, it’s such an easy capacity to build; all you need is a good brain and a computer.
What I envision for the future, and part of my Bill and Melinda Gates Foundation grant is dedicated to this, is creating a network of modellers in the Mekong region working on questions from their own countries and interacting with their own governments in order to support public health.
Part of our efforts in this area have involved establishing, TDModNet, a global network to connect modellers and help overcome the challenges of working in isolation. In my personal experience of the network, there are already success stories. Sheetal Silal and I got to know each other through the network and I ultimately got to co-supervise her modelling PhD; she’s already forming her own research group. Sheetal’s now connected Akindeh Nji who’s received TDModNet travelling award to work with her in South Africa. Akindeh’s background is in the medical statistics area and has very close collaborations with the NMCP in his home country, Cameroon. It’s almost certain that his new skills and research will be fast-tracked into policy. That’s just one example. And, that’s the pleasure for me of working in an area like that – I like the speed at which scientific results get transformed into policy.
To learn more about MAEMOD, visit: http://www.tropmedres.ac/mathematical-economic-modelling
To learn more about TDModNet, visit: http://www.tdmod.net/